What Is Your Favorite Data? A Guide To Identifying And Utilizing Valuable Information
What Is Or Was Your Favorite Data refers to the most valuable or preferred types of information an individual or organization possesses. For instance, a retail company may consider customer purchase history as their favorite data, as it provides insights into buying patterns.
Favorite data is crucial for decision-making, research, and strategic planning. It enables organizations to understand trends, predict outcomes, and optimize operations. Historically, the rise of big data has significantly increased the volume and variety of favorite data available, allowing for more comprehensive analysis and insights.
This article will delve into the characteristics, applications, and ethical considerations surrounding favorite data, providing valuable insights for businesses and individuals seeking to harness its power effectively.
Read also:Rose Scott Nude Separating Facts From Fiction
What Is Or Was Your Favorite Data
Identifying and utilizing favorite data effectively requires a comprehensive understanding of its essential aspects. These include:
- Accuracy: Data's freedom from errors and distortions.
- Completeness: Data's inclusion of all necessary information.
- Consistency: Data's conformity to defined standards and formats.
- Currency: Data's up-to-dateness and timeliness.
- Granularity: Data's level of detail and specificity.
- Relevancy: Data's alignment with the specific needs and goals.
- Security: Data's protection from unauthorized access or disclosure.
- Timeliness: Data's availability when it is needed.
- Uniqueness: Data's distinctiveness and value compared to other data.
- Volume: Data's amount and quantity.
Understanding these aspects enables organizations to effectively leverage favorite data for informed decision-making, strategic planning, and operational optimization. It also helps ensure data's reliability, usability, and alignment with business objectives.
Accuracy
Accuracy, defined as data's freedom from errors and distortions, plays a crucial role in determining the value and usability of favorite data. Inaccurate data can lead to flawed decision-making, biased analysis, and misinformed conclusions. Conversely, accurate data provides a solid foundation for reliable insights, effective planning, and optimal outcomes.
For instance, consider a healthcare organization that relies on patient data to make treatment decisions. If the data contains errors or inconsistencies, it could result in incorrect diagnoses, inappropriate treatments, and adverse patient outcomes. Similarly, in the financial sector, inaccurate data can lead to faulty risk assessments, misguided investments, and substantial financial losses.
Ensuring the accuracy of favorite data requires implementing robust data quality practices throughout the data lifecycle. This includes data validation, verification, and regular audits to identify and correct errors. Organizations must also establish clear data governance policies and standards to maintain data integrity and consistency. By prioritizing accuracy, organizations can maximize the value of their favorite data and mitigate the risks associated with inaccurate information.
Completeness
Within the realm of favorite data, completeness stands as a critical aspect, ensuring that data encompasses all essential information to provide a comprehensive and reliable foundation for analysis and decision-making.
Read also:Chiara Mazzola Nude The Truth Behind The Clickbait
- Attribute Completeness
Attribute completeness refers to the inclusion of all relevant attributes or features for each data point. For instance, in a customer database, ensuring the presence of attributes such as name, contact information, and purchase history contributes to attribute completeness. - Record Completeness
Record completeness pertains to the inclusion of all necessary records within a dataset. In the context of sales data, this means having a complete record of all transactions, including details such as product purchased, quantity, and price. - Temporal Completeness
Temporal completeness focuses on the inclusion of data across the entire relevant time period. For instance, in tracking website traffic, ensuring the availability of data for all days and hours provides temporal completeness. - Contextual Completeness
Contextual completeness involves the inclusion of additional information that provides context and meaning to the data. For example, in analyzing customer feedback, including the context of the feedback (e.g., product or service being reviewed) enhances contextual completeness.
Achieving completeness in favorite data is crucial as it helps organizations gain a holistic understanding of the data, make informed decisions, and derive meaningful insights. Incomplete data can lead to biased analysis, inaccurate conclusions, and suboptimal decision-making. By ensuring completeness, organizations can unlock the full potential of their favorite data and drive better outcomes.
Consistency
Within the realm of favorite data, consistency emerges as a fundamental pillar, ensuring that data adheres to predefined standards and formats. This conformity plays a pivotal role in guaranteeing the reliability, usability, and effective analysis of data.
Consistency eliminates ambiguity and ensures uniformity in data representation, enabling seamless integration, comparison, and aggregation from diverse sources. It facilitates accurate data interpretation, meaningful insights, and informed decision-making. Inconsistent data, on the other hand, can lead to errors, misinterpretations, and flawed conclusions.
Real-life examples abound, highlighting the critical role of consistency in favorite data. In financial reporting, adherence to standardized accounting principles ensures consistency in financial data, enabling accurate comparisons and reliable analysis. Similarly, in scientific research, consistency in experimental protocols and data recording methods allows for the replication of results and the validity of findings.
Practical applications of this understanding are vast. By establishing and enforcing data consistency standards, organizations can improve data quality, streamline data management processes, and enhance collaboration and data sharing. This, in turn, leads to better decision-making, more efficient operations, and a competitive advantage in data-driven industries.
In conclusion, consistency is an indispensable component of favorite data, underpinning its reliability, usability, and value. By ensuring consistency, organizations can unlock the full potential of their data, driving informed decision-making, optimizing operations, and achieving their strategic objectives.
Currency
Currency, referring to data's up-to-dateness and timeliness, stands as a critical component of favorite data. It ensures that data reflects the most recent and accurate information, providing a solid foundation for informed decision-making and effective operations.
Consider a scenario where an e-commerce company relies on customer purchase data to analyze buying patterns and optimize inventory levels. If the data is not current, the company may make decisions based on outdated information, leading to missed opportunities, overstocking, or stockouts. In contrast, up-to-date data provides a real-time view of customer behavior, enabling the company to make agile adjustments to its inventory strategy and maximize sales.
Another example is in the healthcare industry, where timely access to patient data is crucial for accurate diagnosis and effective treatment. Outdated medical records can lead to delays in diagnosis, inappropriate treatment decisions, and adverse patient outcomes. Currency in healthcare data ensures that clinicians have the most recent information at their fingertips, allowing them to make informed decisions and provide optimal care.
Organizations can ensure currency in their favorite data by implementing automated data refresh processes, establishing regular data update schedules, and leveraging real-time data collection technologies. By prioritizing currency, organizations can gain a competitive edge, improve operational efficiency, and deliver better outcomes.
Granularity
Within the realm of favorite data, granularity emerges as a critical aspect, referring to the level of detail and specificity present within the data. It plays a significant role in determining the usability, interpretability, and actionable insights that can be derived from the data.
- Attribute Granularity
Attribute granularity pertains to the level of detail captured for each attribute or characteristic of data. For instance, customer data can be collected with varying levels of granularity, ranging from basic demographics to detailed purchase history and behavioral patterns. - Record Granularity
Record granularity focuses on the level of detail captured for each individual record within a dataset. For example, sales data can be recorded at the transaction level, providing granular details of each purchase, or at a higher level, such as daily or weekly sales. - Temporal Granularity
Temporal granularity refers to the level of detail captured over time. Data can be recorded at various time intervals, ranging from real-time streaming to hourly, daily, or monthly snapshots. The appropriate temporal granularity depends on the nature of the data and the analysis objectives. - Contextual Granularity
Contextual granularity encompasses the level of detail captured about the context surrounding the data. This includes information such as the source of the data, any transformations or manipulations performed, and the environment in which the data was collected.
Granularity is a crucial consideration in favorite data as it affects data analysis, decision-making, and the overall value derived from the data. By carefully defining the appropriate level of granularity, organizations can ensure that their data meets their specific needs and supports informed decision-making. Striking the right balance between granularity and data volume is essential for maximizing the value of favorite data.
Relevancy
Within the realm of favorite data, relevancy takes center stage, referring to the data's alignment with the specific needs and objectives of an organization or individual. This aspect plays a crucial role in determining the value and actionable insights that can be derived from the data.
- Business Objectives
Relevancy ensures that the data collected and analyzed is directly tied to the strategic objectives of the organization. This alignment enables data-driven decision-making that supports business goals, such as increasing revenue, optimizing operations, or enhancing customer satisfaction. - Target Audience
Understanding the target audience is essential for determining data relevancy. Data that is relevant to one group may not be relevant to another. For instance, customer purchase data is highly relevant for marketing campaigns targeting existing customers but may be less relevant for product development initiatives. - Decision-Making
Relevancy is directly linked to effective decision-making. By focusing on data that is relevant to the decision at hand, organizations can make informed choices that are tailored to their specific context and needs. This enhances the quality of decision-making and leads to improved outcomes. - Competitive Advantage
In today's data-driven business landscape, relevancy is a key differentiator. Organizations that can leverage relevant data to gain insights into customer behavior, market trends, and competitive dynamics can gain a competitive edge and drive superior performance.
By carefully considering the relevancy of favorite data, organizations can maximize its value, make informed decisions, and achieve their strategic objectives. Relevancy ensures that data is not just a collection of numbers but a powerful tool that drives business success.
Security
Within the realm of favorite data, security emerges as a paramount aspect, safeguarding data from unauthorized access or disclosure. This protection is crucial for preserving the confidentiality, integrity, and availability of data, ensuring its value and usability.
- Access Control
Access control mechanisms, such as authentication and authorization, restrict who can access and manipulate data. This prevents unauthorized individuals from gaining access to sensitive information, protecting data from theft or misuse. - Encryption
Encryption involves converting data into an encoded format that can only be decrypted by authorized parties. This measure ensures data confidentiality, especially when it is transmitted or stored in insecure environments. - Data Masking
Data masking techniques obscure sensitive data by replacing it with fictitious or synthetic values. This helps protect data privacy while still allowing it to be used for analysis or testing purposes. - Audit Trails
Audit trails record all access and modification attempts made to data. These logs provide a detailed history of data usage and help detect unauthorized access or data breaches.
By implementing robust security measures, organizations can safeguard their favorite data from unauthorized access or disclosure. This protection ensures data confidentiality, integrity, and availability, enabling organizations to leverage their data effectively while minimizing risks and maintaining compliance with data protection regulations.
Timeliness
Within the realm of favorite data, timeliness emerges as a crucial aspect, ensuring that data is readily available when it is needed for decision-making, analysis, and operations. Timeliness plays a pivotal role in maximizing the value of data and enabling organizations to respond swiftly to changing market dynamics and customer demands.
- Real-Time Data
Real-time data refers to data that is available as soon as it is generated or captured. This immediacy is critical in scenarios where real-time decision-making is essential, such as fraud detection, traffic management, and online customer engagement. - Near Real-Time Data
Near real-time data is data that is available with minimal delay, typically within seconds or minutes of being generated. This level of timeliness is valuable for applications that require rapid response times but do not demand the absolute immediacy of real-time data, such as inventory management and supply chain optimization. - Batch Data
Batch data is data that is collected and processed periodically, such as daily or weekly. While not as timely as real-time or near real-time data, batch data is still valuable for analysis and reporting purposes, providing insights into trends and patterns over longer time frames. - Historical Data
Historical data refers to data that has been collected and stored over a period of time. This data is essential for trend analysis, forecasting, and understanding the evolution of business metrics. Historical data provides a valuable context for current data and helps organizations make informed decisions based on past performance and industry trends.
By understanding the different facets of timeliness and aligning data availability with their specific needs, organizations can harness the full potential of their favorite data. Timeliness ensures that data is actionable, relevant, and supports data-driven decision-making in a rapidly changing business environment.
Uniqueness
Within the landscape of favorite data, uniqueness emerges as a critical differentiator, determining its distinctiveness and value compared to other data. This uniqueness stems from the inherent characteristics of the data, its rarity, and its ability to provide novel insights and competitive advantages.
Uniqueness is often a direct consequence of the specific context and domain from which the data is derived. For instance, customer loyalty data collected by a retail company may be unique due to the company's unique customer base, loyalty program structure, and market positioning. This uniqueness makes the data valuable for understanding customer behavior, optimizing marketing campaigns, and personalizing customer experiences.
Real-life examples abound, showcasing the practical significance of uniqueness in favorite data. In the healthcare industry, genomic data is highly unique due to the inherent variability of individual genetic profiles. This uniqueness enables personalized medicine, allowing healthcare providers to tailor treatments and therapies based on a patient's specific genetic makeup, improving patient outcomes and reducing trial-and-error approaches.
The practical applications of understanding the uniqueness of favorite data are vast. By identifying and leveraging unique data assets, organizations can gain a competitive edge, make informed decisions, and drive innovation. This understanding helps prioritize data collection efforts, optimize data storage and management strategies, and develop tailored data analysis techniques to extract maximum value from unique data.
Volume
Within the realm of favorite data, volume emerges as a critical aspect, referring to the sheer amount and quantity of data available. The volume of data has a profound impact on the value and usability of data, presenting both opportunities and challenges for organizations.
- Data Size
Data size refers to the physical size of the data in terms of storage space. As data volumes grow, organizations must invest in scalable and cost-effective data storage solutions to accommodate the influx of data. - Data Velocity
Data velocity refers to the rate at which data is generated and collected. The increasing velocity of data, driven by real-time applications and IoT devices, poses challenges for data processing and analysis. - Data Variety
Data variety refers to the different types and formats of data available. The proliferation of unstructured data, such as text, images, and videos, presents challenges for data integration and analysis. - Data Redundancy
Data redundancy refers to the duplication of data across multiple systems or sources. Redundancy can lead to data inconsistencies and challenges in data management and analysis.
Understanding the volume of favorite data is crucial for organizations to effectively manage, analyze, and derive value from their data. By addressing the challenges associated with data volume, organizations can harness the full potential of their data and gain a competitive edge in the data-driven economy.
In conclusion, our exploration of "What Is Or Was Your Favorite Data" has provided valuable insights into the characteristics, applications, and ethical considerations surrounding this critical asset. Key points highlighted throughout this article include the importance of data accuracy, completeness, consistency, currency, granularity, relevancy, security, timeliness, uniqueness, and volume. These aspects are interconnected and interdependent, forming a holistic framework for understanding and leveraging favorite data effectively.
As organizations navigate the ever-evolving data landscape, it is imperative to prioritize favorite data as a strategic asset. By investing in data quality, governance, and analytics capabilities, organizations can unlock the full potential of their data, drive informed decision-making, and gain a competitive advantage. The insights gained from this article serve as a foundation for continuous improvement and innovation in the realm of data management and utilization.


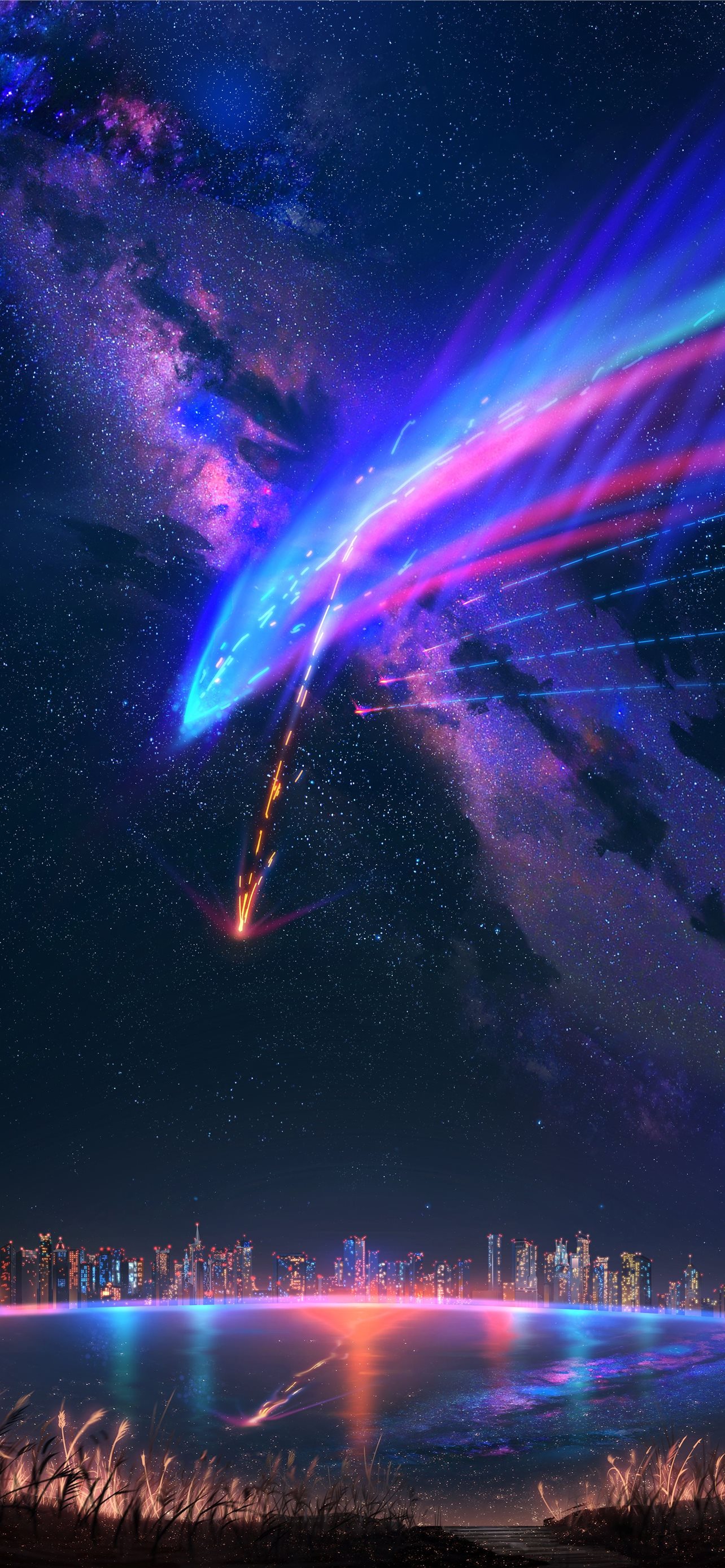
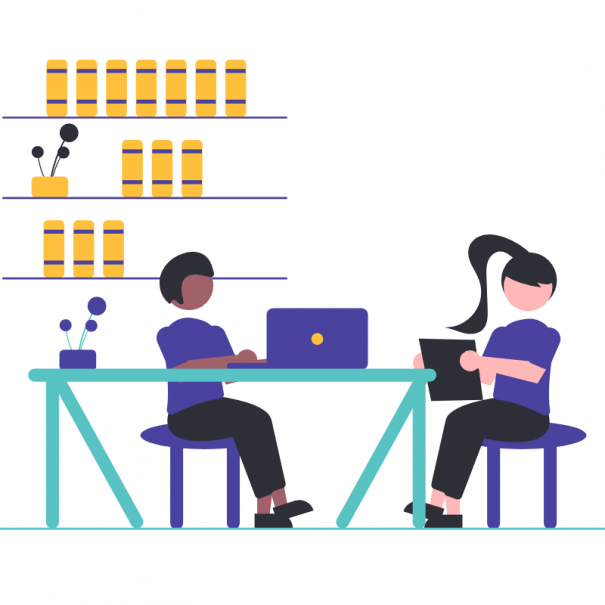